10 Top Steps to Get Started with a Data Pipeline With AI
In the era of Artificial Intelligence (AI), the prowess of data has become a strategic asset for businesses. Crafting a robust data pipeline is pivotal for organizations aspiring to harness the full potential of AI. At Greys Essex IT Services
11/18/20232 min read
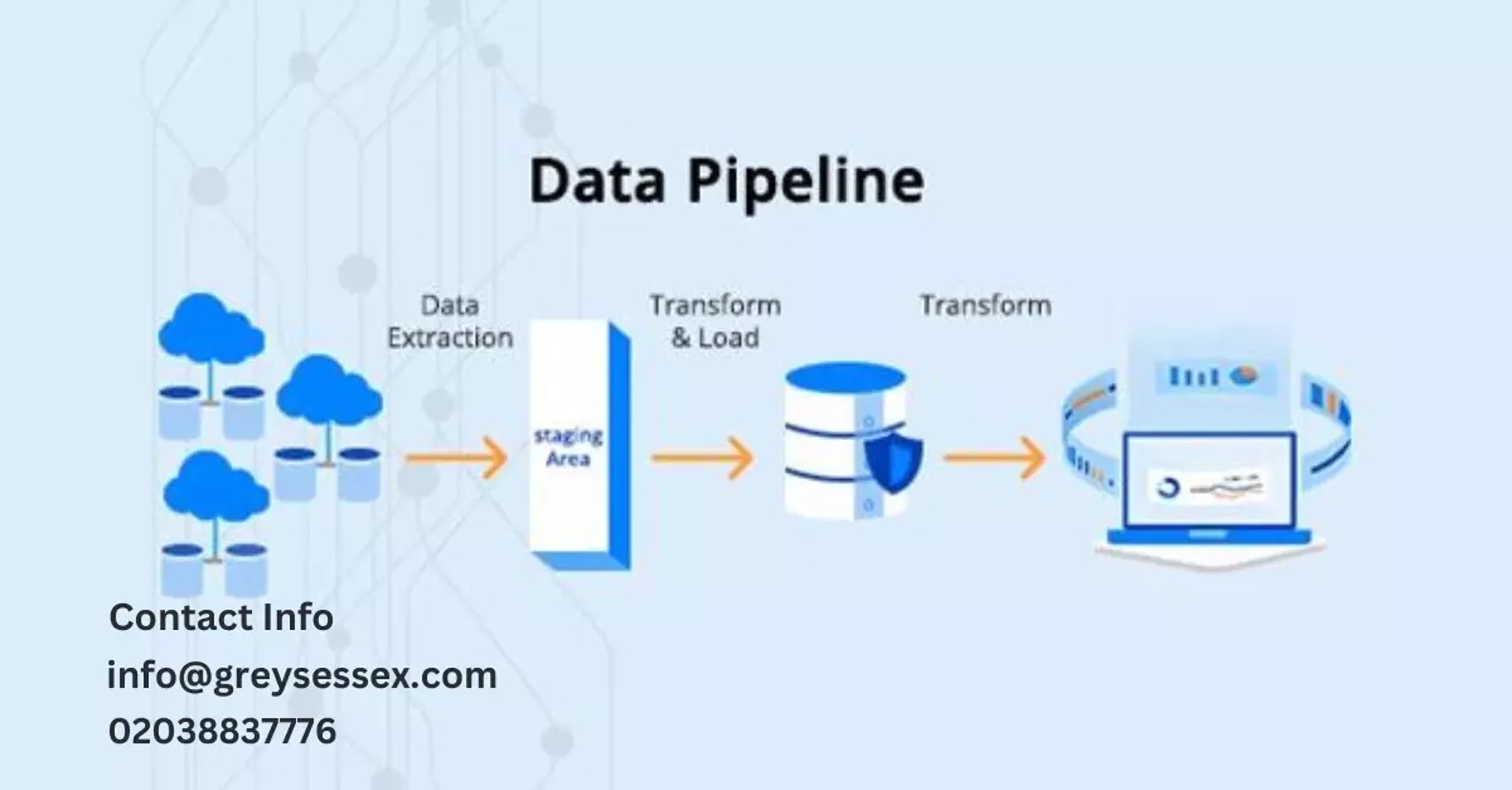
Data prowess has become a strategic asset for businesses in the age of Artificial Intelligence (AI). Building a strong data pipeline is critical for organisations looking to fully realise the potential of AI. We recognise the transformative role that a well-architected data pipeline plays in unleashing the power of AI at Greys Essex IT Services. In this blog, we will walk through the top steps for getting started with a data pipeline designed for AI integration.
1. Outline Your Goals:
Begin by clearly outlining your goals. Determine which AI applications you intend to deploy and the insights you hope to gain from data.
2. Assess Data Sources:
Conduct a comprehensive assessment of your data sources. Whether it's structured or unstructured, understanding the nature of your data is fundamental to pipeline design.
3. Data Quality Assurance:
Implement stringent data quality assurance measures. Ensure that your data is accurate, complete, and consistent to derive meaningful insights.
4. Choose Appropriate Tools:
Select tools that are appropriate for your data processing and analysis needs. Consider things like scalability, compatibility, and integration ease.
5. Data Integration:
Combine multiple data sources into a unified platform. This stage entails harmonizing data formats, resolving inconsistencies, and creating a unified flow.
6. Put Data Storage in Place:
Choose a data storage solution that meets your volume and retrieval speed requirements. Relational databases, NoSQL databases, and cloud-based storage are all options.
7. Data Processing Frameworks:
Make use of robust data processing frameworks such as Apache Spark or Hadoop. These frameworks make distributed processing of large datasets possible.
8. Integration of Machine Learning:
Machine learning frameworks should be integrated into your pipeline. This step entails deploying models, fine-tuning algorithms, and ensuring data infrastructure compatibility.
9. Continuous Monitoring:
Implement a robust monitoring system to track the health and performance of your data pipeline. This includes real-time monitoring and alerts for potential issues.
10. Iterative Optimization:
Data pipelines are dynamic systems that require iterative optimisation. Iterate and optimize your pipeline in response to changing business needs, technological advancements, and data landscapes.
Greys Essex's Competitive Advantage:
Greys Essex IT Services specializes in customizing data solutions that integrate seamlessly with AI initiatives. Our expertise is in orchestrating end-to-end data pipelines that enable organisations to make confident data-driven decisions.
Conclusion
Finally, for businesses seeking a competitive advantage, embarking on the journey of creating a data pipeline for AI is a strategic imperative. Greys Essex helps organisations navigate these transformative steps, ensuring that their data becomes an enabler for AI-driven success. With Greys Essex, embrace the future of AI and realise the full potential of your data.
Our Social Accounts:
Location
Aziz Bhatti Shaheed Road, Chishti Mall, Model Town A, Bahawalpur, Pakistan
Hours
I-V 9:00-18:00
VI - VII Closed
Contacts
+92 303 0859841
info@greysessex.com
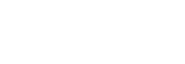
